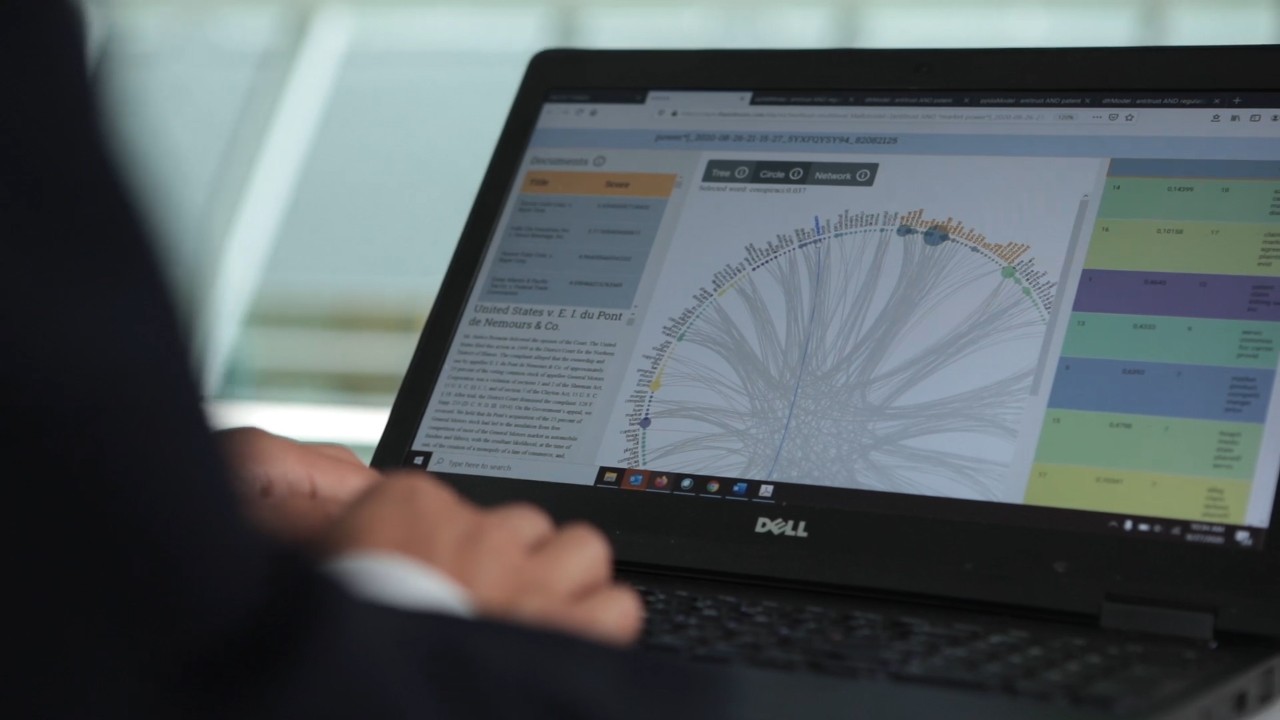
UC researchers use data analysis to tackle issues in antitrust
Question of how federal courts approach regulation, antitrust leads to collaboration
Professor Felix Chang, associate dean for Faculty and co-director of the Corporate Law Center at Cincinnati Law, wanted to understand how federal courts approach topics in antitrust such as market power, or the balance between antitrust and regulation. The challenge was, in order to do this, he would need to process large amounts of data in the form of tens of thousands of federal cases. This dilemma led to a collaboration with the Digital Scholarship Center at the University of Cincinnati. They used machine learning to understand how federal courts think through these complex topics in antitrust.
Read on for a Q&A with Professor Chang about how this collaboration came about, what they've discovered, and where they hope this research will go next.
Tell us about the topic modeling research you’re doing with the Digital Scholarship Center. How did this project come about?
This is a collaboration I’ve undertaken with the Digital Scholarship Center, which is part of the library system at the University of Cincinnati. The library system has secured a million dollar grant from the Andrew W. Mellon Foundation to do big data analysis, and in particular, it’s using algorithmic topic modeling (a form of natural language processing) to go through very large data sets.
The College of Law at the University of Cincinnati is one of the strategic partners that the Digital Scholarship Center has identified, and so we've explored this algorithmic topic modeling by analyzing a really large data set of antitrust cases. I wanted to see what topic modeling illuminates, for instance, on the market power doctrine, or the balance between antitrust and regulation.
How did you come to this method for analyzing case law? Did the questions precede the methods, or vice versa?
I was not very familiar with big data analysis and I wasn't familiar with topic modeling at all. It was through the introduction of our librarian, Jim Hart, that I started to partner with the Digital Scholarship Center at UC.
I came to him with this problem, which is, we've got very large amounts of data—really, cases—and how can we think about how federal courts approach squishy topics in antitrust doctrine such as market power and the balance between antitrust and regulation. And it was actually through Jim Hart that he introduced me to others on main campus who were at the university's library system and working on topic modeling.
What sorts of concepts or questions have these methods been useful for exploring as it relates to the work you’re doing around antitrust and related concepts?
Topic modeling is a mechanism that abides by this concept of distant reading (whereas in law we're very used to close reading texts). Because I was new to this concept, I began very conservatively and just wanted to unleash that mechanism to see what trends came up. So, I came to it with no preconceptions whatsoever, other than thinking that federal courts probably aren't very good at pinning down what market power means; also, they probably aren't very precise about the balance between antitrust and regulation.
We kind of let topic modeling go. These algorithms work best as a form of unstructured natural language processing over large and unstructured datasets, and with the copious amounts of natural language, really case law, I wanted to see what patterns were illuminated.
As a research method in legal scholarship, is topic modeling something many other scholars are doing? Is it controversial? Disruptive?
The use of topic modeling has been happening in legal academia, but I would say that we're still kind of at the infancy of the use of topic modeling in law. I can think of precedents among academics. Michael Livermore, for instance, has a series of papers looking at judicial style using topic modeling. Before that there were others. David Law, now at the University of Virginia, has used topic modeling to look at patterns in constitutions around the world.
A lot of topic modeling's first uses were as more of an informational retrieval mechanism. It was used, for instance, at the Stanford dissertation browser to try to see patterns in dissertations that had been uploaded onto the dissertation browser. Some early uses also included using topic modeling to recommend scientific articles.
It’s oftentimes the case that, among academics, we tend to be very siloed in our approach. When we cite other works, we tend to cite highly cited papers. And highly cited papers tend to come within our disciplines. But the thing is that across disciplines, many people are looking at the same problems, but from a variety of different perspectives. What topic modeling does is cut to the key words. It is able to create visual descriptions of the relationships, the statistical relationships, among words, and individually map out the likelihood that these words will tend to cluster together into topics. Topic modelling then, can tell you that across disciplines, if you've got a few key words, for instance, climate change, for instance, antitrust and market power, or antitrust and regulation, here are how the different disciplines are looking at these concepts.
Can you walk through any conclusions or findings your research has helped surface?
Professor Felix Chang
Our colleagues in the Digital Scholarship Center have built this platform where you can upload large datasets. The dataset that we've selected is all federal cases bearing either the word “antitrust” or “regulation” from Harvard Law Library’s Case Law Access Project. We uploaded hundreds of thousands of cases bearing these keywords, and we've separated them into different corpora. For the market power corpus, that is, all federal antitrust cases with the words “market power,” we began to see some key patterns. We saw some key patterns also for what I call the antitrust regulation corpus, that is, all federal cases bearing the words “antitrust” and “regulation.”
Topic modeling is really good at showing you the big picture, some of the large patterns. It’s really interesting because some of the large patterns that we've seen are really a diversification of the types of cases that you see in market power cases, or when the balance between antitrust and regulation is litigated. We see a diversification of cases, and simultaneously you see a decline of certain cases. It seems, for instance, that a lot of tying cases aren’t being brought as much, and in their place, you're seeing a lot more of what I think of as litigation-centric topics, so topics dealing with antitrust, civil procedure or the various stages of civil litigation and a lot of class action cases as well.
I think that the big picture you see over time is a diversification of antitrust cases. I think that complicates a lot of previous research, which empirically was oftentimes discussing whether antitrust cases have declined over time or not. I think the picture that emerges is probably one of greater nuance.
Have you seen downsides or weaknesses in applying this methodology to legal research?
Where I think we should be cautious is in drawing inferences. Here I'm probably a little bit more conservative about topic modeling because I'm pretty new to it.
In a number of the papers that I began to co-write with the Digital Scholarship Center, I lay out where I think the weaknesses are for topic modeling. In particular, we've been aware for a long time that one of the critiques in digital humanities is that this sort of distant reading mechanism can oftentimes obscure context. Because what you're doing is you're splicing words, you're taking them out of context to try to illuminate patterns that are very broad and big picture patterns, but that can only be done if you take them out of context.
Now, that said, our partners at the Digital Scholarship Center have tried to give us technical fixes. For instance, they will run a visualization multiple times over multiple iterations to try to preempt what we call the wobble, or variation in results. The platform that the Digital Scholarship Center has created also has this information retrieval or document retrieval function where you can actually pull up cases and read through them to make sure that they cohere with the topic.
Are there wider implications to employing these research methods in legal scholarship?
Where I think this is going that's particularly interesting is, we're going to be very transparent about our methodology and about our results. I hope that ultimately this will push the proprietary legal research services to be more forthcoming as well. Scholars have pointed out before that Lexis and Westlaw and some of these other commercial databases are not very clear about how their algorithms work, about how their algorithms pull cases, for instance.
This is particularly interesting and important because we're at a juncture in which the market for legal information is diversifying. You are seeing insurgents challenge the incumbents. You have a lot of new legal databases that are promising disruption through algorithms that can grab cases more accurately. And so, as we're in this moment of inflection, my hope is that through this partnership with the Digital Scholarship Center, we can push a lot of these legaltech and incumbent commercial legal databases to be much more forthcoming and transparent about how their own algorithms work.
There’s obviously an interdisciplinary element to this—both in the methods and the research on antitrust. Is this part of a larger trend in legal academia? How has it impacted your work?
Law has become much more interdisciplinary and certain subsets of legal scholarship are much more interdisciplinary than others. But computational legal analysis in particular is really exciting for what it portends in the way of partnerships between legal academics and computer scientists, and also legal academics and digital humanists.
A lot of these mechanisms such as topic modeling originated from computer sciences. And their earliest application was in digital humanities. And I actually think it's great that we're working together.
Now, the area that I tend to write in, financial regulation and antitrust, you tend to see a lot more interdisciplinary collaboration anyway. We're really used to working with economists. So I'm really excited about what we can do with topic modeling. Because I think that, for instance, antitrust is already very used to partnering in law and economics. I think it's a natural extension to say, let's partner with some digital humanists and computer scientists to think about novel and innovative ways to look at large amounts of legal texts.
Author: Nick Ruma
Photo Credit: Andrew Higley/UC Photography
Related Stories
University of Cincinnati celebrates DAAP's class of 2024:...
April 27, 2024
Discover the achievements of the University of Cincinnati's College of Design, Architecture, Art, and Planning's Class of 2024 as they graduate into the alumni family, showcasing exceptional talent and innovation. From prestigious awards to prominent job offers, these graduates exemplify the transformative power of creativity and dedication in shaping tomorrow's leaders.
CCM shares 2024 alumni and distinguished service awards
April 26, 2024
UC's College-Conservatory of Music is proud to present alumni and distinguished service awards to outstanding members of our community at the end of each academic year. The 2024 awards are presented to four accomplished alumni and one dedicated patron in a private virtual event on April 23.
OTR mural centerpiece of 'big' celebration of UC alumni
April 26, 2024
New downtown artwork salutes 18 alumni award recipients who personify UC’s alumni success.